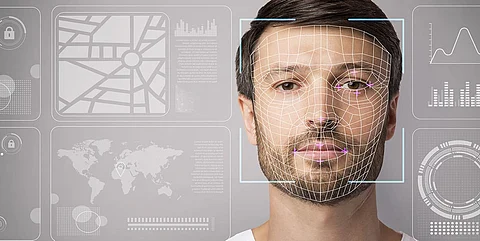
Deepfake Technpology
Deepfake Technology you need to know about
Deepfakes technology refers as deep learning artificial intelligence to substitute the image of one person in the video as well as other digital media with that of another. Computers are becoming increasingly capable of mimicking reality. The modern film, for example, depends significantly on computer-generated settings, scenery, and individuals in place of the realistic settings and props that were historically prevalent, and these sequences are often indistinguishable from reality.
Deepfake technology has recently made the news. Deepfakes are the most recent generation of computer imaging, formed when artificial intelligence (AI) is designed to substitute one person's face with another in recorded video.
Deepfake Techology
The name "deep fake" is derived from the underlying technology "deep learning," which is a type of artificial intelligence. Deep learning algorithms, which utilize massive amounts of data to train themselves how to solve issues, are used to replace faces in the video and digital content to create realistic-looking false media.
There are numerous techniques for making deep fakes, but the most frequent is to utilize deep neural networks with autoencoders that use a face-swapping methodology. You'll need a target video to serve as the foundation for the deepfake, followed by a series of video clips of the person you wish to insert into the target.
The videos can be entirely unrelated; for example, the target could be a clip from a Hollywood film, while the films of the person you wish to integrate into the film could be random clips grabbed from YouTube.
The autoencoder is a deep learning AI software that is tasked with analyzing the video clips to comprehend what the person looks like from various perspectives and environmental situations, and then mapping that person onto the human in the target video by identifying common traits.
Another form of machine learning, known as Generative Adversarial Networks (GANs), is added to the mix, which finds and fixes any errors in the deepfake across numerous rounds, making it more difficult for deepfake detectors to decode them.
GANs are also a common way for developing deepfakes, depending on the analysis of massive quantities of data to "learn" how to create new instances that imitate the actual thing, with frighteningly exact results.
Many experts predict that as technology advances, deepfakes will become significantly more sophisticated, posing more substantial concerns to the public, such as election meddling, political unrest, and increased criminal activities.
How Deepfake Technology used?
While the capacity to automatically swap faces to generate genuine and realistic-looking synthetic video has some intriguing benign uses (such as in film and gaming), it is a hazardous technology with some problematic applications. Deepfakes were used to make synthetic pornography, which was one of the earliest real-world uses.
In 2017, a Reddit user titled "deepfakes" developed a porn forum using face-swapped actors. Since then, porn (especially revenge porn) has frequently made headlines, severely sullying the reputations of celebrities and public people. According to a Deeptrace analysis, pornography accounted for 96% of deepfake films discovered online in 2019.
Deepfake video has been utilized in politics as well. In 2018, for example, a Belgian political party produced a video of Donald Trump delivering a speech in which he called on Belgium to withdraw from the Paris climate accord. Trump, on the other hand, never gave the speech; it was a ruse. That was not the first time a deepfake was used to generate deceptive films, and tech-savvy political analysts are preparing for a forthcoming wave of fake news that includes impressively realistic deepfakes.
Advancement in Deepfake Technology
Deepfakes aren't only confined to videos. Deepfake audio is a rapidly expanding field with several uses. Realistic audio deepfakes can now be created using deep learning algorithms with as little as a few hours (or, in some cases, minutes) of the voice of the person whose audio is being cloned, and once a model of a voice is created, that person can be made to say anything, as was the case last year when the fake sound of a CEO was used to commit fraud.
Deepfake audio has medical uses in the original voice replacement, as well as in computer video games - now programmers may allow in-gamer characters to say anything in real-time, rather than depending on a restricted set of scripts produced before the game was released.
As deepfakes grow more popular, society will most likely need to adjust to identifying deepfake videos in the same way that internet consumers have become accustomed to detecting other types of fake news. In many cases, like in cybersecurity, more deep fake technology needs to arise to identify and prevent it from spreading, which may set off a vicious cycle and perhaps do even more harm.
Deepfakes can be identified by a few indicators:
· Current deepfakes have difficulty animating faces convincingly, resulting in videos in which the person never blinks, or blinks far too frequently or unnaturally. However, once University of Albany researchers published a paper revealing the blinking irregularity, fresh deepfakes were produced that no longer had this problem.
· Look for skin or hair issues, as well as faces that appear to be blurrier than the surroundings in which they are placed. The focus may appear abnormally soft.
· Is the illumination artificial? Deepfake algorithms frequently keep the lighting from the clips used as prototypes for the fake video, which would be a poor match for the brightness in the target video.
· The audio may not appear to fit the person, particularly if the video was fabricated but the actual audio was not as expertly edited.